Programming machines to display intelligent actions is what artificial intelligence is all about.
Predictions indicate that the artificial intelligence market would develop at a CAGR of 37.3% between 2023 and 2030.
Forbes
It’s easy to understand why artificial intelligence is essential.
Let us dig into the following parts to learn more about AI systems and examine some relevant AI examples:
What is Artificial Intelligence (AI)?
An AI system is a computer that can perform tasks similar to those done by humans and other animals. This includes functions like perceiving, analyzing, and making inferences.
On the other hand, AI examples of such artificial intelligence applications include voice recognition, image processing, language translation, and different input mappings.
AI examples range from –
- Web search engines such as Google Search,
- Recommendation systems utilized by YouTube, Amazon, and Netflix are highly advanced,
- Ability to understand human speech like Siri and Alexa,
- Self-driving cars like Waymo,
- AI-powered tools can generate creative outputs like ChatGPT and AI art,
- They can also excel at the highest level in strategic games like chess and Go.
The AI system impact refers to the trend of formerly regarded “intelligent” jobs becoming less so as computers improve.
One of the most famous AI instances, OCR, is seldom mentioned.
Since its 1956 founding, artificial intelligence has gone through cycles of promise, disillusionment, and budget cutbacks.
Which are followed by fresh starts, breakthroughs, and fresh investments.
AI systems have failed through simulating the brain, modeling human problem-solving, formal reasoning, massive data volumes, and replicating animal behavior.
Highly mathematical and statistical machine learning was the norm in the first decade of the 21st century.
The comprehensive tool allows academics and practitioners in numerous domains to overcome previously intractable challenges.
There are many types of AI application research, each with its aims and methods.
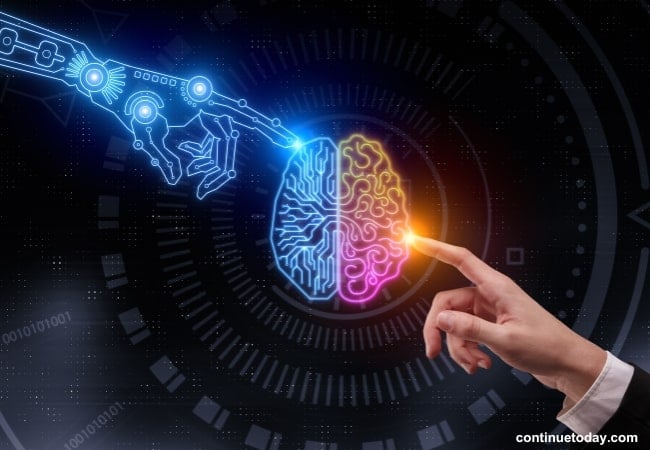
Research in artificial intelligence (AI) has traditionally focused on several areas, including-
- Reasoning,
- Knowledge Representation,
- Planning,
- Learning,
- Natural Language Processing,
- Vision,
- Movement,
- Manipulation of Objects and so on.
One of the ultimate aims of the profession is general intelligence, or the capacity to answer any issue.
Researchers working on AI systems have adapted and incorporated many different approaches to problem-solving.
Such as search and mathematical optimization, formal logic, artificial neural networks, and methodologies based on statistics, probability, and economics to address these challenges.
AI applications use many fields of study besides computer science, psychology, languages, philosophy, and philosophy.
The field’s central assumption is that human intellect “may be so precisely defined that a computer may be developed to replicate it.”
This sparked ethical and philosophical debates about the implications of constructing artificial creatures with human-level intellect.
Topics that have been studied in myth, literature (including science fiction), and philosophy since antiquity.
Many philosophers and computer scientists have cautioned that AI systems represent a grave danger to humanity.
Especially if we do not harness their reasoning capability for good.
Some have argued that the term “artificial intelligence” (AI) exaggerates the capabilities of AI technology.
History of Artificial Intelligence Technology
Mary Shelley’s Frankenstein and Karel Apek’s R.U.R. employ intelligent artificial beings.
These people’s struggles predicted numerous AI systems’ ethical debates.
Ancient philosophers and mathematicians were the first to investigate what we now call “mechanical” or “formal” reasoning.
Alan Turing’s theory of computing directly resulted from studying mathematical logic.
Which postulated that a computer could imitate any act of logical deduction by rearranging symbols as elementary as “0” and “1.”
The Church-Turing thesis states that digital computers may mimic any formal reasoning.
Due to these, researchers began to investigate the feasibility of creating an electronic brain.
Also, other developments in neuroscience, information theory, and cybernetics occurred around the same time.

In 1943
The first AI system was McCullouch and Pitts’ Turing-complete “artificial neurons” formal design.
In 1950
By this time, two competing theories on how to make AI systems practical had arisen.
The Symbolic AI system, also known as GOFAI, was an attempt to utilize computers to build a symbolic representation of the world.
Also, a set of systems that could reason about the world.
Allies included Herbert A. Simon, Marvin Minsky, and Herbert Newell.
The “heuristic search” theory is closely related to this one.
It views intelligence as a problem of searching through a set of hypotheses to find the right one.
The second vision, connectionism, aimed to develop artificial intelligence via study and practice.
The leading proponent of this strategy was Frank Rosenblatt.
He wanted to model the Perceptron connections after the connections between neurons.
James Manyika and others have drawn parallels between connectionist AI and the way humans think.
Symbolic methods, according to Manyika, were the most prominent in the early 20th-century drive for AI systems.
Because of their ties to the philosophical canons of thinkers like Descartes, Boole, Gottlob Frege, Bertrand Russell, and others.
Cybernetics and other connectionist artificial neural networks have gained prominence in recent decades.
It was during a workshop held in 1956 at Dartmouth College that the study of AI’s practical AI applications began.
Attendees established themselves as pioneers and industry leaders in artificial intelligence.
Their “astonishing” systems allowed computers to learn checkers strategies, solve algebra word problems, establish logical theorems, and speak English.
In 1960
During this period, laboratories were established worldwide, and the United States government heavily invested in research.
In the 1960s and 1970s, researchers were confident that symbolic techniques would lead to the development of an AI system capable of performing complex tasks.
Herbert Simon predicted that machines would be capable of performing every task a human can within the next two decades.
“Within a decade… the difficulty of building ‘artificial intelligence’ will basically be addressed,” declared Marvin Minsky.
They needed to appreciate the magnitude of the challenges that remained.
In 1974
After facing criticism from Sir James Lighthill and persistent pressure from the US Congress to finance more productive programs.
Since the US and UK halted exploratory research in AI, its advancement has stagnated. An “AI winter” followed, making it hard to fund AI system projects.
In 1980
Expert systems, a kind of AI application program that mimics human experts’ knowledge and analytical abilities.
Those were commercially successful during this period, reviving research into AI system development.
In 1985
This year, the market for AI systems surpassed $1 billion, and the public began to gain familiarity with AI applications case studies.
Simultaneously, the Japanese government’s fifth-generation computer project prompted the U.S. and British governments to increase money for research.
In 1987
Nonetheless, starting with the Lisp Machine market collapse in 1987, AI systems again fell into contempt, ushering in a second, more protracted winter.
Some scientists started questioning whether the symbolic method could successfully replicate all aspects of human cognition.
It includes vision, robotics, learning, and pattern recognition.
Several scientists started investigating “sub-symbolic” methods for solving specific AI application-related issues.
Researchers in robotics, like the late Rodney Brooks, turned their attention away from symbolic AI technologies.
Towards the fundamental technical challenges of allowing robots to move, survive, and learn from their surroundings.
The mid-1980s saw a resurgence of interest in neural networks and “connectionism,” a term coined by Geoffrey Hinton and David Rumelhart.
Neural networks, fuzzy systems, Grey system theory, evolutionary computation, and many more technologies.
Those derived from statistics or mathematical optimization emerged as essential soft computing components in the 1980s.
AI technology regained its reputation in the late 1990s and early 2000s by successfully solving specific challenges.
Researchers were able to create conclusions that could be independently verified and make more use of mathematical techniques.
Also work in tandem with experts from other disciplines (including statistics, economics, and mathematics) because of the right emphasis.
In 2000
Even though the term “artificial intelligence” was seldom used to characterize the solutions.
They were produced by developers of AI systems in the 1990s and were extensively employed by the year 2000.
In 2012
Data-hungry deep learning approaches began to dominate accuracy standards in about 2012.
Faster processors, improved algorithms, and access to vast quantities of data all contributed to progress in machine learning and perception in 2012.
In 2015
Jack Clark of Bloomberg claims that 2015 was a watershed year for AI applications.
With over 2,700 software projects using AI technology at Google, up from “sporadic use” in 2012.
He said this was because of improvements in cloud computing that allowed for cheaper neural networks, as well as better research methods and larger data sets.
In 2017
Twenty-five percent of businesses have “integrated AI technology in their services or operations,” according to a poll.
Research on AI systems has increased by 50% from 2015 to 2019, as indicated by the total number of publications.
Academics worried that AI applications were diverging from their fundamental mission of developing general-purpose, human-level intelligence in computers.
Statistics-based AI solutions, including highly effective methods like deep learning, are central to most of the present research effort.
As a result of this worry, the area of artificial general intelligence (or “AGI”) emerged. By the 2010s, it was home to numerous well-endowed institutions.
Current Situation
The computer scientist Jaron Lanier presented an alternate perspective on AI systems in The New Yorker in April 2023, arguing that they are not as clever as their name and popular culture would have you believe.
Lanier summarizes his article by saying, “Consider the human race. Humans are the key to solving the difficulties of digital data.
Goals of AI Technology
The challenge of artificial intelligence applications simulation has been decomposed into its parts.
These features and skills that scientists have determined must be present in an intelligent system.
The characteristics listed below are the ones that have garnered the most significant interest.
- Deductive Thinking and Issue Solving
- Data Representation
- Learning Process
- Language Comprehension
- Perception Building
- Social Relationships
- Standard Intelligence
Deductive Thinking and Issue Solving

It was the goal of the first academics to create algorithms that could mimic the kind of logical deduction and problem-solving that humans are capable of.
Using ideas from probability and economics, researchers in artificial intelligence applications developed strategies for handling incomplete or ambiguous data in the 1980s and 1990s.
The “combinatorial explosion” that plagued many of these algorithms rendered them incapable of effectively addressing substantial reasoning challenges.
As the scale of the issues increased, their response time slowed dramatically.
The step-by-step deduction, which early AI system research could mimic, is seldom used even by humans.
They make snap decisions and use intuition to solve most issues.
Data Representation

Knowledge representation, common sense knowledge, description logic, and ontology are some primary articles here.
Knowledge is represented in an ontology as a collection of ideas and their interrelationships that are specific to a certain area.
By representing engineering knowledge, AI technology may provide insightful responses to inquiries and draw inferences from data.
An ontology is a formal description of “what is” software agents can understand that. It contains the following: a set of objects; a set of relations; a set of concepts; and a set of qualities.
Upper ontologies are the most general ontologies, and they try to provide a basis for all other information by mediating between domain ontologies, which encompass specialized knowledge about a particular knowledge topic.
To be completely intelligent, a computer program must have access to common knowledge.
Ontologies’ semantics are commonly expressed using description logic like the Web Ontology Language.
Tools developed from real AI applications depict objects, features, and classifications.
It also learned to make connections between things like situations; events; states and time; causes and effects; knowledge, default reasoning (things that humans assume to be true until told otherwise and will remain valid even when other facts are changing); and so on.
Most people’s knowledge is not in the form of “facts” or “statements,” it’s sub-symbolic knowledge.
AI faces a challenge in dealing with the vast amount of common knowledge people hold.
Content-based indexing and retrieval, scene interpretation, clinical decision support, knowledge discovery, and other AI applications use formal knowledge representations.
Learning Process

In artificial intelligence applications, machine learning (ML) refers to the study of algorithms that learn and become better on their own.
Using a stream of data as input, unsupervised learning may identify patterns and be one of the perfect AI examples.
Classification and numerical regression are the two most common types of supervised learning, which involve human labeling of the input data.
The algorithm learns to classify new inputs by viewing AI examples of them in several categories.
Regression is the process of finding a function that characterizes and predicts the change in outputs as a function of changes in inputs.
Classifiers and regression learners may be considered “function approximators” that attempt to learn an unknown (potentially implicit) function.
In AI examples, a spam classifier is a function that learns to categorize emails as either “spam” or “not spam” based on their content.
To train an agent, reinforcement learning systems incentivize desirable behaviors while discouraging undesirable ones. To solve its difficulty, the agent sorts its observations into categories.
Knowledge obtained from solving one issue may be used to solve a different problem, a process known as transfer learning.
Computational learning theory uses sample complexity (how much data is required) or optimization techniques to assess learners.
Language Comprehension

It is now possible for machines to comprehend human language, thanks to the advancements in natural language processing (NLP).
With a robust natural language processing system, we can learn from human-written sources like newswire stories and leverage natural-language user interfaces.
However, simple uses of natural language processing include search engines, FAQ databases, and translation software.
Symbolic artificial intelligence uses formal syntax to translate the underlying structure of phrases logically.
Due to the complexity of logic and the breadth of common sense, this did not lead to any practical AI applications.
Additionally, examples of modern statistical methods include-
- Calculation of co-occurrence rates (the frequency with which two words appear in close proximity to one another),
- “Keyword spotting” (the process of looking for a certain term to retrieve information),
- Transformer-based deep learning (the process of identifying patterns in text), and others.
By 2019, they will be able to produce text with sufficient precision for use on web pages or in documents.
Perception Building

Cameras, microphones, wireless communications, active lidar, sonar, radar, and tactile sensors are all AI examples of sensors contributing to machine perception.
Computer vision has numerous applications, including facial recognition, object identification, and voice recognition.
Moreover, its primary focus is on processing and analyzing visual data.
Social Relationships

Systems that can detect, analyze, process, or mimic human affective states fall under the umbrella of affective computing, a multidisciplinary field.
Some AI virtual assistants talk in a conversational or even humorous tone to seem more sensitive to human emotions.
However, this might mislead naive users into thinking that current computer agents are far more sophisticated than they are.
Affective computing has had some limited success with AI applications like textual sentiment analysis and, more recently, multimodal sentiment analysis.
Whereby artificial intelligence software categorizes the effects presented by a filmed subject.
Standard Intelligence

A computer with general intelligence can solve on a scale and with a degree of flexibility comparable to that of the human mind.
Several different approaches have been proposed for creating AGI, or artificial general intelligence.
According to Hans Moravec and Marvin Minsky, a high-level multi-agent system or cognitive architecture with general intelligence may combine the efforts of specialists in several fields.
In order to achieve AGI, Pedro Domingos believes there must be a “master algorithm” that is theoretically simple yet technically challenging.
Some people think that anthropomorphic elements, such as a fully functional artificial brain or a simulation of childhood development, would eventually lead to the emergence of general intelligence.
The Tools Needed for an AI System
Optimizing the AI examples requires a specialized set of tools.
What follows is a description of the resources needed for a more profound comprehension:
- Optimizing Searches
- Logic
- Probability Theory for Uncertainty
- Predictive Models and Other Statistical Learning Techniques
- Synthetic Neural Systems
- Deep Learning
- Domain-specific Software and Hardware
Optimizing Searches

AI can scan and pick the best option through a process of elimination.
One way to think about logical proof is by applying inference rules to a set of premises to arrive at a group of conclusions.
Through a process known as means-ends analysis, planning algorithms explore possible paths toward a given goal by traversing a hierarchy of intermediate objectives.
Algorithms used in robotics for manipulating limbs and gripping items do local searches in configuration space.
Also, the search space rapidly becomes so large that simple exhaustive searches are usually insufficient for solving real-world issues.
Moreover, the resulting search is unreasonably sluggish or fails altogether.
Pruning the search tree is a technique used in various search algorithms where heuristics exclude options with a low likelihood of success.
The “best estimate” for the solution route is provided to the program via heuristics. With the use of heuristics, we can narrow down the number of possible answers.
However, a new sort of search, grounded on optimization theory, gained popularity in the 1990s.
For many issues, an initial assumption may be refined in small steps until no more improvements can be realized in the search.
Genomic algorithms, expression programming, and genetic programming are all AI examples of traditional evolutionary algorithms.
Another option is using swarm intelligence algorithms to coordinate dispersed searches.
Particle swarm optimization (motivated by bird flocking) and ant colony optimization (motivated by ant trails) are two standard swarm algorithms used in the search.
Logic

Knowledge representation and problem-solving are two common uses of logic, but many more areas exist.
The sat plan algorithm, as an AI example, employs logic for planning, while inductive logic programming is a learning technique.
The study of artificial intelligence makes use of many distinct kinds of reasoning.
Also, “or” and “not” are examples of truth functions used in propositional logic.
The use of quantifiers and predicates allows first-order logic to describe facts about objects, their attributes, and their relationships to one another.
To propositions like “Alice is old” (or “Alice is wealthy,” “Alice is tall,” or “Alice is hungry”) that are too ambiguous to be definitively true or untrue, fuzzy logic applies a “degree of truth” (between 0 and 1) to them.
Circumscription, default logic, and non-monotonic logic help with default reasoning and the qualifying problem.
Description logics, event calculus, fluent calculus (for events and time), causal calculus, belief calculus (belief revision), and modal logic are some logic extensions for specialized knowledge.
In addition, logic like paraconsistent logic has been developed to simulate inconsistent claims in multi-agent systems.
Probability Theory for Uncertainty

The agent must often make decisions with little or ambiguous data in order to solve issues in artificial intelligence (such as thinking, planning, learning, perception, and robotics).
Using techniques from probability theory and economics, academics working on AI systems have developed a variety of tools to address these issues.
The Bayesian inference algorithm, the expectation-maximization algorithm, and the planning algorithms of decision networks.
Also, the perceptual algorithms of dynamic Bayesian networks all fall under the umbrella of Bayesian networks as a reasonably generic tool.
To improve perception systems, use hidden Markov models and Kalman filters. They filter, predict, smooth, and clarify data streams.
Utility, a fundamental notion in economics, quantifies the worth of a good or service to a rational actor.
Decision theory, decision analysis, and the theory of information value are three precise mathematical techniques established to examine how an agent might choose and plan.
Methodologies like game theory, mechanism design, and Markov decision processes are also part of this toolkit.
Predictive Models and Other Statistical Learning Techniques

Classifiers (“if shining, then diamond”) and controllers (“if diamond, then pick it up!”) are the two most basic forms of artificial intelligence.
However, controllers also categorize situations prior to inferring actions; hence, classification is often at the heart of artificial intelligence systems.
Functions known as classifiers employ pattern matching to identify the most promising candidates.
They are very desirable for use in artificial intelligence systems due to their adaptability via example tuning.
These AI examples of artificial intelligence applications’ functionality are observations or patterns.
Each pattern in supervised learning is assigned to a certain category.
Choosing a class is required.
A data set consists of all the observations and the labels for each category.
Each time a new observation is received, it is evaluated based on how closely it resembles previous statements.
There are multiple statistical and machine-learning methods available to train a classifier.
One of the most used symbolic machine learning algorithms is the decision tree.
Until the middle of the 1990s, the k-nearest neighbor algorithm was the most popular analogical AI system.
In the 1990s, kernel approaches such as the support vector machine (SVM) supplanted the k-nearest neighbor.
Because of its scalability, the naive Bayes classifier is said to be Google’s “most extensively used learner.”
The process of classifying data also makes use of neural networks and notable AI examples.
Classifier effectiveness is sensitive to data features such as dataset size, sample distribution across classes, complexity, and noise.
If the presumed model is an excellent match for the real data, model-based classifiers do well.
SVM classifiers are more precise than naive Bayes classifiers for fundamental data analysis.
Which provided that no matching model is available and accuracy (rather than speed or scalability) is the primary priority.
Synthetic Neural Systems

The structure of human brain neurons served as a model for the development of neural networks.
A primary “neuron” N takes “votes” on whether or not to activate from other neurons, each of which, when “fired,” has an equal but opposite effect.
One basic technique (called “fire together, wire together”) for learning involves increasing the weight of two linked neurons when the activation of one stimulates the successful activation of another.
Also, neurons can process information nonlinearly, rather than just counting votes, and have a continuous spectrum of activation.
Modern neural networks have become indispensable to represent complicated interactions between inputs and outputs and discover patterns in data.
They are capable of studying discrete logical procedures and even continuous functions.
During training, a neural network creates a multi-dimensional topology, which may be thought of as a mathematical optimization problem.
Backpropagation is the most widely used training algorithm.
For neural networks, other learning strategies include GMDH, competitive learning, and Hebbian learning (“fire together, connect together”).
Recurrent neural networks provide feedback and short-term memory of earlier input events, and acyclic, or feedforward, neural networks, in which the information flows only in one way, are the two basic types of networks.
Perceptrons, multi-layer perceptrons, and radial basis networks are all AI examples of feedforward networks.
Deep Learning

Between the network’s inputs and outputs, deep learning employs many layers of neurons. Layers of processing power allow for more refined feature extraction from initial data.
In image processing, as AI examples, lower layers may be responsible for identifying boundaries, whereas higher layers would be responsible for recognizing concepts important to a person, such as numerals, characters, faces, etc.
Many significant areas of artificial intelligence have seen huge improvements in program performance thanks to the advent of deep learning.
Artificial intelligence examples include things like computer vision, voice recognition, picture categorization, and more.
Moreover, several or even all of the layers of a deep learning system may be convolutional neural networks.
Each neuron in a convolutional layer gets information from a small region of the layer below it, referred to as the neuron’s receptive field.
This generates a hierarchy amongst neurons, which is analogous to the structure of the visual cortex in animals, and may drastically decrease the number of weighted connections between them.
An RNN is a deep learning example since the signal will “replay” across each network layer several times.
The vanishing gradient problem occurs while back-propagating long-term gradients in gradient descent-trained RNNs.
The long short-term memory (LSTM) method is effective in avoiding this scenario.
Domain-specific Software and Hardware

The field of artificial intelligence has spawned its own set of specialized languages.
Lisp, Prologue, TensorFlow, and many more are all AI examples of languages used in artificial intelligence.
AI hardware development has seen the introduction of neuromorphic computing and AI accelerators.
The Utilization of Artificial Intelligence
Any mental endeavor may benefit from the use of AI.
The various AI methods used now would take too long to mention individually.
The term “AI effect” is used to explain the tendency for an approach to lose its “AI” label once it gains widespread acceptance.
In the 2010s, artificial intelligence applications were fundamental to the most economically successful sectors of computing, and Nowadays, they have become a crucial component of contemporary living.
Virtual assistants (like Siri or Alexa), autonomous vehicles (drones, ADAS, and self-driving cars), automatic language translation (Microsoft Translate, Google Translate), and facial recognition (Apple’s Face ID) are just some of the many AI applications.
Thousands of AI applications have been developed and deployed successfully to address challenges faced by various sectors of the economy.
Some AI applications include battery storage, deep fake detection, medical diagnostics, military logistics, foreign policy, and supply chain management.

Some Historical Utilization of AI Systems
Artificial intelligence (AI) systems have been put to the test in the realm of gaming since the 1950s.
On 11 May 1997, Deep Blue made history as the first computer chess system to defeat the reigning world chess champion, Garry Kasparov.
Watson, IBM’s question-answering system, soundly beat the greatest Jeopardy! Champions, Brad Rutter and Ken Jennings, in an exhibition match in 2011.
When pitted against Go champion Lee Sedol in March 2016, AlphaGo won four out of five games, making history as the first computer Go-playing system to defeat a professional Go player without using any kind of advantage.
Pluribus and Cepheus are two such programs that can handle imperfect-information AI examples games like Poker at a superhuman level.
In the 2010s, DeepMind created a “general artificial intelligence” capable of picking up a wide variety of Atari games on its own.
In the year 2020, Natural Language Processing systems such as the massive GPT-3 (then by far the biggest artificial neural network) were able to equal human performance on pre-existing benchmarks while not having achieved a commonsense grasp of the contents of the benchmarks.
Tools for artificial intelligence (AI) system content detection analyze digital material, including text, photos, and videos, in order to identify certain categories of content.
Spam, grammatical problems in speech, sexually explicit imagery, and other forms of offensive material are often detected using such programs.
Improved speed and accuracy in identifying unsuitable material, enhanced user safety and security, and decreased legal and reputational concerns.
Especially for websites and platforms are just some of the advantages of employing content detector tools built on AI systems.
Computerized Traffic Signals

Carnegie Mellon has been working on smart traffic signals since 2009.
Since then, Professor Stephen Smith has founded Surtrac, an organization that has deployed intelligent transport systems to 22 different municipalities.
The average installation cost is about $20,000 per junction.
When implemented at busy junctions, it cuts travel times by 25% and reduces traffic wait times by 40%.
Significant AI Examples
The influence of AI on our everyday lives is substantial, yet most of the time, we utilize AI examples without even realizing it.
Let us learn about some well-known AI examples to better grasp the concept.
- Directions and Maps
- Recognition of the Face
- Editing Documents for Spelling and Grammar
- Search and Result Algorithms
- Chatbots
- Digital Alliances
- Virtual Social Interaction
- E-Payments
Directions and Maps
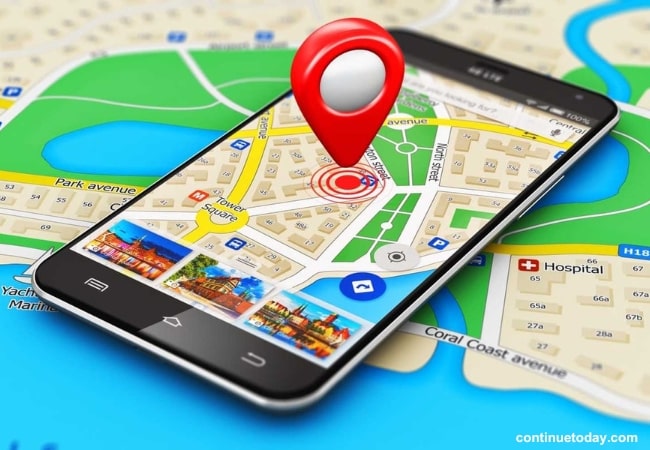
One of the best AI examples is the mapping technology that has made air travel so much easier.
You can now go exactly where you need to go by pulling out your phone, opening Google or Apple Maps, and entering your destination.
So, why does the project set goals in the first place?
What could be better than the best possible route, difficulties, and congestion?
Once reliant on GPS satellite systems, users now have better options because of AI applications.
Algorithms memorize building margins to improve map rendering and house and block number decoding.
The software may also identify shifting traffic patterns to help you avoid backups.
Recognition of the Face

Some of the most ubiquitous AI examples are the facial recognition systems we now rely on to unlock our phones and the virtual filters we apply to our photos.
With the use of face detection, the former can recognize any human face.
In the second scenario, facial recognition may be used to verify the identification of the subject.
Face recognition technology is used for security and surveillance purposes at government buildings and airports.
These AI examples should now be immediately relatable to your own life and work, right?
Editing Documents for Spelling and Grammar

Perhaps you used Grammarly to check over your senior thesis before turning it in.
You may now use it to check the spelling of an email you send to your employer.
That is only one more common AI example, by the way.
To improve the written communication of its users, artificial intelligence systems utilize machine learning, deep learning, and natural language processing to rectify grammatical and spelling errors.
Machines are being educated in grammar by linguists and computer scientists, just as you were.
Since the editor’s algorithms have been trained on high-quality linguistic data, it will detect comma problems in your subsequent draughts.
Search and Result Algorithms

Have you found that recommendations for films and products online seem to be relevant to your tastes and search history?
These highly developed recommendation algorithms study your online behavior to figure out what you like.
Also, you should be aware of this as another excellent AI example.
Both ML and DL collect and analyze data from the user’s front end.
After analyzing your preferences, it may suggest new music or products for you to check out.
Chatbots

Customers could become frustrated with customer care representatives.
In most businesses, it is prohibitively costly, difficult to administer, and underperforming.
The use of artificially intelligent chatbots to address this issue is gaining traction.
Using these algorithms, computers can manage frequently asked questions, take and track orders, and redirect phone calls.
Natural language processing (NLP) allows chatbots to pass for human customer support representatives. Yes/no questions are only one kind of inquiry that chatbots can answer.
They are adept at addressing difficult inquiries.
In fact, the bot will figure out what went wrong if your answer receives a low rating and fix it for the following time, guaranteeing that you will always obtain a perfect score.
Digital Alliances

When we are swamped, we turn to our digital assistants for help.
While you are behind the wheel, have your helper phone your mum.
The digital assistant Siri can search through your contacts, recognize the name “Mom,” and dial your mother’s number.
The helpers determine what you want and use NLP, ML, SAS, and AlgoExec to attempt to get it for you. In practice, voice search is quite similar to image search.
Even artificial intelligence (AI) is utilized in the Metaverse to design and oversee things like NPCs, VAs, and chatbots.
Virtual Social Interaction

Social networking apps are increasingly utilizing artificial intelligence (AI) to rate content, suggest friends, and target advertisements.
Using techniques like phrase analysis and picture recognition, AI systems can quickly identify and remove content that violates terms of service.
Although not entirely, this approach makes use of deep learning neural network architecture.
As a result, social media platforms use AI to facilitate communication between their users and the advertisers and marketers that place the highest value on their profiles.
Social media AI may eventually figure out what kinds of content people like and start recommending it to them.
E-Payments

Since regular banking trips are no longer necessary thanks to AI, you have not been there in over five years.
Using AI, banks are streamlining the payment process for their clients.
You may open an account, transfer funds, or deposit cash from anywhere in the globe thanks to AI-powered security, identity management, and privacy controls.
The purchases made with a credit card might point to fraudulent activity.
Computer science shows even this. Based on the user’s purchasing habits, the frequency of those habits, and the retail and delivery costs, an algorithm may estimate the user’s total spending.
If the system detects activity that does not match the user’s profile, it may issue a warning or request further verification before allowing the transaction to go through.
Protection of AI Works
While the Internet of Things (IoT) is expected to have the highest market size, WIPO indicated artificial intelligence (AI) as the most prolific developing technology.
Basically, in terms of patent applications and issued patents in 2019.
Following IoT, big data technologies, robots, artificial intelligence, 3D printing, and 5G mobile services have become major players in the market.
After the advent of AI in the 1950s, entrepreneurs submitted 340,000 patent applications.
Also, scholars have published 1.6 million scholarly articles on the topic. The vast bulk of AI-related patent filings have been made after 2013.
Of the top 30 AI applications of patents, 26 are companies, and 4 are universities or public research organizations.
There has been a marked movement from theoretical research to the use of AI technology in commercial goods and services.
The ratio of publications to innovations dropped from 8:1 to 3:1 between 2010 and 2016.
In 2016, 134,777 machine learning patents were submitted out of a total of 167,038 AI patents issued in 2016.
Making machine learning the most prevalent AI technology revealed in patents. The most prominent functional application of machine learning is computer vision.
In addition to describing specific AI-related methods and uses, patents in this area often identify a specific industry or sector of use.
In 2016, the top 20 applications were telecommunications (15%), transportation (15%), life and medical sciences (12%), personal devices, computing, and human-computer interaction (11%).
Finance, entertainment, security, industry and manufacturing, agriculture, and networks (including social networks, smart cities, and the IoT) were covered.
IBM’s 8,290 AI applications for the patent are far and by the most numerous, with Microsoft’s 5,930 applications coming in a distant second.
Philosophy of AI

AI theory covers a lot of ground. Let’s delve into more details about it in the paragraphs that follow.
In search of a definition of AI, Alan Turing posed the topic of whether or not computers could reason in a 1950 paper.
He suggested rephrasing the inquiry to focus on the feasibility of intelligent machine behavior rather than on whether or not a machine “thinks.”
The Turing test, which evaluates a computer’s capacity to have a conversation in a human-like fashion, was created by him.
Since the machine’s actions are all we can witness, it makes little difference whether it is “really” thinking or literally has a “mind.”
According to Turing, it is best to assume that everyone is considerate.
In line with Turing, Russell, and Norvig argue that an operational definition of AI is necessary.
However, they are concerned that the exam unfairly pits robots against humans.
They argued that the purpose of aeronautical engineering is not to create “machines that fly so perfectly like pigeons that they can trick other birds.”
John McCarthy, a pioneer in the field of artificial intelligence, concurred, noting, “Artificial intelligence is not, by definition, an imitation of human intellect.”
The computational aspect of the capacity to attain objectives in the world is what McCarthy means by intelligence.
Similarly, “the capacity to solve complex problems” is how Marvin Minsky, another pioneer in the field of AI, characterizes it.
According to these ideas, intelligence entails solving concrete, bounded issues.
This is a definition that Google, a leading AI firm, has embraced as well.
Like the concept of intelligence in biological intelligence, this one specified that the manifestation of intelligence is the capacity of systems to synthesize information.
Assessing AI Methods
For the vast majority of its existence, research into artificial intelligence has been largely unguided by any kind of prevailing unifying theory or paradigm.
In the 2010s, statistical machine learning achieved extraordinary success, overshadowing all other methods (to the point that some sources, notably in the business sector, interpret the phrase “artificial intelligence” to denote “machine learning using neural networks”).
This viewpoint is mostly literal, tidy, gentle, and restricted.
Some people have pointed out that future generations of AI researchers may have to reexamine these problems.
Constrained Artificial Symbolic Intelligence

Symbolic AI (or “GOFAI”) attempted to recreate the kind of abstract, reflective thinking that humans use when doing things like solving puzzles, expressing legal reasoning, or doing maths.
They excelled at “intellectual” activities like mathematics and IQ exams.
A physical symbol system contains the necessary and sufficient means of broad, intelligent action,” Newell and Simon wrote in the 1960s.
However, the symbolic method fell short on many problems that humans readily handle, like learning, object recognition, and commonsense reasoning.
Moravec’s paradox describes AI’s ability to execute high-level “intelligent” tasks but not low-level “instinctive” ones.
Hubert Dreyfus, a philosopher, has maintained since the 1960s that human skill relies on inborn propensities rather than logical analysis, on “feel” rather than explicit symbolic knowledge, and on the manipulation of symbols rather than conscious instinct.
Artificial intelligence (AI) research later agreed with him despite first mocking and ignoring his claims.
We still have not found a solution since sub-symbolic thinking is prone to the same kinds of opaque errors that intuition is, including algorithmic bias.
Sub-symbolic AI is a step away from explainable AI, which is why critics like Noam Chomsky claim that more research into symbolic AI will still be essential to achieve universal intelligence.
Understanding why a sophisticated statistical AI program made a choice may be challenging, if not impossible.
The developing discipline of neuro-symbolic AI makes an effort to unite the two schools of thought.
Neat vs. Scruffy
Intelligent behavior, “neats” believe, can be summed up in a few well-defined concepts (such as logic, optimization, or neural networks).
“Scruffies” believe it needs to resolve a plethora of unconnected issues (particularly using common sense thinking).
Russell and Norvig called this shift, which occurred in the 1990s, “the triumph of the neats,” and it was the result of the widespread adoption of mathematical techniques and rigorous scientific standards that had been controversial in the preceding decades.
Soft vs. Hard Computing
Many significant situations present a challenge in finding a solution that is provably right or optimum.
Tolerance for approximate results, fuzziness, ambiguity, and partial truth are hallmarks of soft computing methods, including evolutionary algorithms, fuzzy logic, and neural networks.
With the advent of neural networks in the late 1980s, soft computing emerged as the foundation for the majority of successful artificial intelligence programs in the 21st century.
Narrow vs. General AI
There is a debate among AI experts as to whether the field should focus on developing broad artificial intelligence and superintelligence (general AI) or narrow AI.
Which aims to solve as many issues as possible in the hopes that doing so may eventually lead to the field’s ultimate objectives.
Modern AI has had more verifiable accomplishments by concentrating on particular issues and specialized solutions, and this is because general intelligence is hard to define and quantify.
This is the exclusive focus of the experimental branch of AGI, or artificial general intelligence.
Artificial Intelligence, Cognition, and Awareness

The field of philosophy of mind is uncertain as to whether or not a computer may possess mental qualities analogous to those of a human person.
Instead of focusing on the machine’s exterior behavior, this problem examines the machine’s inside experiences.
Most researchers in the area of artificial intelligence dismiss this concern since it does not have any bearing on their overall objectives.
Stuart Russell and Peter Norvig’s research on AI reveals that many researchers prioritize program functionality over ethical considerations.
They do not care whether it is fake intelligence or the actual thing.
However, this inquiry has recently emerged as a major topic in the study of the mind.
It is also the fundamental problem with artificial intelligence in most fictional works.
Consciousness
David Chalmers classified the challenges of contemplating the mind as “hard” and “easy,” respectively.
Deciphering the brain’s signal processing, planning, and behavioral control mechanisms is a simple challenge.
The challenge is in articulating what this sensation resembles or why it exists at all.
It is simple to describe how humans absorb information but far more challenging to explain how they view the world subjectively.
A colorblind individual who has trained himself to recognize red things in their environment is a useful illustration of artificial intelligence in action.
However, it is unclear what information the individual would need to recognize the color red.
Computationalism and Functionalism
The computationalism is a school of thought in the philosophy of mind that holds that mental processes are like computations performed by a computer.
Computationalism proposes a solution to the mind-body conflict by asserting that the connection between the brain and the rest of the body is analogous to that between computer programs and their physical implementations.
Philosophers Jerry Fodor and Hilary Putnam first presented this view in the 1960s, and they drew inspiration from the work of AI researchers and cognitive scientists at the time.
John Searle, a philosopher, coined the term “Strong AI” to describe this view: Computers can have minds like humans with proper design and inputs/outputs.
The Chinese room argument is Searle’s rebuttal to this claim; it aims to demonstrate that even if a computer successfully mimics human behavior, there is no reason to assume it likewise has a consciousness.
Legal Protections for Robots

If a computer can think and feel, it is possible that it has sentience (the capacity to feel) and that it may experience pain; if so, it deserves protection under the law.
Rights for hypothetical robots would fall somewhere between those for animals and those for humans.
Despite being a topic of discussion in fiction for centuries and being studied by organizations like the California Institute for the Future, some critics argue that the subject is premature.
Progress in Artificial Intelligence
Given how extensively we use AI now, it’s reasonable to assume that we’ll see countless more examples of it in the future.
Learn more about the most accurate AI system forecast for the future below.
The term “superintelligence” refers to a hypothetical agent with intelligence far above that of even the most brilliant and talented human mind.
The term “superintelligence” may also be used to describe the kind or level of intelligence shown by this kind of agent.
Research into AGI might one day lead to self-improving software that can rewrite its own instructions.

The enhanced program would be even better at enhancing itself, leading to a self-sustaining improvement loop.
An intelligence explosion would cause it to far outpace humans in terms of intellect.
Vernor Vinge, a science fiction writer, used the term “singularity” to describe this event.
Predicting the potential limitations of intelligence or the capabilities of superintelligent computers is a challenging task if not an impossible one. As a result, what occurs after the technological singularity is entirely unknown.
Hans Moravec, a roboticist, Kevin Warwick, a cyberneticist, and Ray Kurzweil, an inventor, have all prophesied that in the future, humans and robots would mix to create cyborgs that are superior to both.
Transhumanism is an ideology with its origins in the work of Aldous Huxley and Robert Ettinger.
The notion that “artificial intelligence is the next step in evolution” was initially presented by Samuel Butler in 1863’s “Darwin Among the Machines.”
And later developed by George Dyson in 1998’s “Artificial Intelligence: A Brief History,” is central to Edward Fredkin’s argument.
Risks of AI System
The aforementioned AI applications make it clear that AI has a considerable effect on our regular job routines.
Despite the many benefits that artificial intelligence has brought about, it does not come without drawbacks.
Let us find out more about them, too –
Lack of Work Due to Technology

Despite technology’s history of increasing employment, economists believe that AI is a “new terrain.”
To determine if AI and robots might raise long-term unemployment, economists were surveyed.
However, they do believe that redistribution of productivity gains using appropriate AI examples may be a net benefit.
For instance, although Michael Osborne and Carl Benedikt Frey claim 47% of American occupations are “high risk” of possible automation, an OECD analysis defines just 9% of American employment as “high risk.”
Many middle-class occupations are at risk of being automated away by AI, unlike in earlier rounds of autonomous AI examples.
The fear that AI applications may do to white-collar occupations what steam power did to blue-collar professions during the Industrial Revolution is “worth taking seriously,” according to The Economist.
Paralegals and fast food chefs are two examples of occupations in danger, whereas the healthcare industry and the ministry both stand to gain from an aging population.
Bad Guys and AI Weapons

Authoritarian regimes may benefit greatly from the AI toolset, which includes smart spyware, facial recognition, and voice recognition, all of which facilitate extensive monitoring.
Such monitoring enables machine learning to identify and categorize prospective adversaries of the state, making it harder for them to remain hidden.
Propaganda and false information spread via recommendation systems may have a much greater impact, and deepfakes facilitate the spread of fake news.
Meanwhile, technological advancements in artificial intelligence are making centralized decision-making competitive with liberal and decentralized systems like markets.
In addition to traditional weaponry, terrorists, criminals, and rogue governments may use weaponized AI applications in the form of sophisticated digital warfare and deadly autonomous weapons.
As of 2015, more than fifty nations were reportedly investigating the potential of combat robots.
Artificial intelligence that learns on its own may also create thousands of potentially dangerous compounds in a couple of hours.
Implicit Bias in Algorithms

Once trained on real-world data, AI systems may develop biases.
Developers are typically unaware of the prejudice since the software learns it. When selecting training data, it is possible to unintentionally introduce bias.
Correlations may also be a source of this: By using AI applications software, we may categorize people into groups and then make predictions about them based on the assumption that they will be similar to others in those categories.
This presumption may not always be fair.
In the United States, as AI examples, judges often utilize a commercial program called COMPAS to predict whether or not a person would re-offend.
ProPublica found that COMPAS overestimates black offenders’ recidivism risk more than white ones.
If marginalized populations are not protected, the many-to-many mapping may affect health equity.
There are currently no equality-focused instruments or laws in place to guarantee the inclusion and utilization of equity AI applications.
Algorithmic bias may potentially lead to unfair outcomes in AI-based credit rating and hiring.
The 2022 ACM Conference on Fairness, Accountability, and Transparency in Seoul, South Korea, presented and published findings.
It advises limiting self-learning neural networks trained on large, unregulated, and imperfect internet data until they are bias-free.
Peril of Extinction

Superintelligent AI applications may potentially enhance themselves to the point that humans could no longer control them.
Stephen Hawking, a scientist, warns that this “spells the end of the human race.”
Philosopher Nick Bostrom believes that intelligent AI systems will exhibit convergent behavior.
Such as accumulating resources or avoiding shutdown, to achieve a goal.
This AI may injure people to reach its final state, which may need more resources or avoidance of shutdown.
Regardless of how modest or “friendly” the intentions behind AI applications may be, he finds that they nevertheless constitute a threat to humanity.
According to political scientist Charles T. Rubin, “Any sufficiently advanced kindness may be indistinguishable from malevolence.”
There is no a priori reason for humans to anticipate that computers or robots will share our theory of morality. Thus, we should not expect them to treat us well.
Experts and businesspeople disagree on the risks of superintelligent AI.
Elon Musk, Stephen Hawking, Bill Gates, and Yuval Noah Harari, all of whom teach history, are among those who have voiced deep concern about AI’s trajectory in the future.
OpenAI and the Future of Life Institute have raised over a billion dollars for ethical AI research.
Artificial intelligence, in its current form, is useful, and it will continue to help people
Mark Zukerberg
Others in the field say that studying the potential dangers is pointless since they are so far off in the future or that humans will be useful to a superintelligent computer.
Some experts, including Rodney Brooks, have predicted that “malevolent” AI is hundreds of years away at the earliest.
Copyright

Decision-making AI raises issues of liability and intellectual property protection for original creations.
Moreover, many different legal systems are working to solve these problems in artificial intelligence.
However, AI-generated works’ copyright protection has been questioned.
The AI System’s Moral Mechanisms
AI applications designed to be friendly prioritize minimizing risks and making choices that benefit humans.
Eliezer Yudkowsky, who coined the term, believes that prioritizing the development of friendly AI applications should be a top research goal.
It is imperative to make a substantial investment beforehand to ensure that AI technology does not become a menace to humanity.
This investment needs to be made before AI poses an existential threat.
Intelligent machines are capable of making ethical decisions by utilizing the principles and procedures of machine ethics.
In 2005, an AAAI symposium established machine morality, computational ethics, or computational morality.
Other methods, such as Wendell Wallach’s “artificial moral agents” and Stuart J. Russell’s three principles, aim to develop machines that are provably beneficial.
AI Policy and Legislation

Policymakers and legislators have been hard at work crafting regulations for AI to ensure safe and effective AI examples of artificial intelligence applications use cases.
This has implications for the more general rules governing algorithms.
A developing problem in jurisdictions throughout the world is the landscape of regulations and policies around artificial intelligence.
More than 30 nations have developed AI-specific policies between 2016 and 2020.
Also, each with its own set of appropriate AI examples.
Canada, China, India, Japan, Mauritius, the Russian Federation, and Several countries plan to implement AI applications on a national scale, including Saudi Arabia, UAE, the US, and Vietnam.
Basically, Bangladesh, Malaysia, and Tunisia were among those working on their own AI strategies.
Launched in June 2020, the Global Partnership on Artificial Intelligence calls for the creation of AI applications.
Mainly in line with human rights and democratic ideals to secure public confidence and trust in the technology.
Notable figures, including Henry Kissinger and Eric Schmidt, called for government oversight of AI systems in a November 2021 declaration.
Science Fiction and Artificial Intelligence
In his 1921 play R.U.R., which stands for “Rossum’s Universal Robots,” Karel Apek popularised the term “robot.”
Ancient literature has depicted artificial beings with free will.
The idea that a human creature might turn against its creators is a recurring theme in these works, which first appeared in Mary Shelley’s Frankenstein.
Fictional AI examples include Arthur C. Clarke’s and Stanley Kubrick’s 1968 2001: A Space Odyssey,
HAL 9000, the murderous computer in charge of the Discovery One spaceship, and 1984’s The Terminator and 1999’s The Matrix,
Also Rare loyal robots like Gort from “The Day the Earth Stood Still” and 1986’s Bishop, and rare human characters like Gort and Bishop.
Among Isaac Asimov’s numerous works, is “The Multivac,” a series about a super-intelligent computer.
It stands out as a prime illustration of Asimov’s use of the Three Laws of Robotics.
Since practically all AI application researchers have heard of Asimov’s rules, thanks to pop culture, they are all acquainted with them.
However, experts in the field of AI examples largely deem them worthless due to a number of factors, including their vagueness.
The science fiction shows Dune and the manga series Ghost in the Shell both examine transhumanism, or the union of people and robots.
Also, several works use AI systems to make us address the basic issue of what it is that makes us human by presenting us with artificial creatures who can feel and hence suffer.
You may find AI examples of this in works as diverse as R.U.R. by Karel Apek, A.I. Artificial Intelligence, and Ex Machina, as well as Philip K. Dick’s classic Do Androids Dream of Electric Sheep?
Dick mulls the possibility that AI-made gadgets change the way we conceptualize human subjectivity.
Final Thoughts
As we approach the future, the number of AI examples seems endless.
Exciting and terrifying in equal measure is the potential of AI to revolutionize our daily lives, whole economies, and entire civilizations.
Every day, AI systems are becoming increasingly common, intelligent, and integrated into our lives.
They help us make better judgments, speed up routine processes, and get access to previously inaccessible areas of information.
However, as we go ahead, we must guarantee that AI examples development is in line with ethical concerns and human values.
Transparent, fair, and responsible AI application development is essential to avoid prejudice, bias, and unintended consequences.
Moreover, there is great potential in working together with machines since it will spur creativity and increase our efficiency.
AI applications are vital to growth, but they carry hazards so we must guarantee that future AI technology benefits everyone.
AI can help with missile launches, difficult medical operations, and email spam filtering. If the public regards AI as unpredictable and untrustworthy, they may be less willing to work with it.
Abused limited AI endangers human health in three ways: by increasing human manipulation and control, by dehumanizing lethal weapons, and by making human work redundant.
Automation is one way AI is altering the future. Advances in machine learning allow computers to do activities formerly reserved for humans. From driving to answering calls, this includes everything.